Credit: Jirsak / Shutterstock
Leadership and AI insights for 2025: The latest from MIT Sloan Management Review
By
The new year is here, and artificial intelligence remains top of mind for many enterprise leaders. The latest guidance from MIT Sloan Management Review explores how best to use generative AI safely and effectively, as well as how to build partnerships that have AI at their foundation. But we begin with something fundamental: a look at what it takes to build strong corporate culture.
Actionable advice for developing a healthy corporate culture
Improving corporate culture is one of the hardest jobs an executive can face. Previous research from MIT Sloan professor of the practice Donald Sull found no correlation between official corporate values and culture, suggesting that leaders are out of touch with the everyday experiences of their employees.
In a new series, Culture Champions, and Charles Sull, the co-founders of research and consulting firm CultureX, share advice from executives who have achieved a track record of business success without sacrificing a positive employee experience. Valuable insights include:
- “You can’t copy and paste values across organizations. ... Employees can sniff out a lack of authenticity. ... You have to be ... clear about who you are.” – Katie Burke, former chief people officer, HubSpot
- “If you say something and people just kind of nod or don’t argue with you, that’s the biggest insult. ... The worst you can do is not garner [some] kind of criticism, pushback, or some degree of debate.” – Jim Whitehurst, former CEO, Red Hat
- “We have a strong belief that our culture is a strategic differentiator to our business strategy. ... We have a high expectation that when you are a leader, a significant amount of your time is spent on the development of your teams.” – Marvin Boakye, chief human resources officer, Cummins
For generative AI in the workplace, governance policies beat bans
Fifteen years ago, IT leaders debated banning personal devices from the corporate network. Today, similar conversations focus on whether to restrict generative AI tools such as ChatGPT in the workplace, given that the technology could introduce security risks along with productivity gains.
To understand the benefits and risks of Bring Your Own AI, and at the MIT Center for Information Systems Research surveyed executives from 50 organizations. Leaders agreed that generative AI restrictions were “neither practical nor effective” and would likely backfire, so they offered five actions that support responsible use of the technology:
- Assemble a cross-functional leadership team to set clear guardrails indicating instances when generative AI tools are acceptable and when they’re too risky.
- Provide hands-on training for identifying proper use cases, communicating with generative AI tools, and thinking critically about the output of AI models.
- Offer a limited number of approved generative AI tools; this helps employees make informed choices and reduces the burden of licensing and maintenance.
- Recognize that measurable short-term ROI may be limited as employees explore new ways of applying generative AI to the way they work.
- Explore how strategic business objectives could benefit from an infusion of generative AI, as well as how individual productivity gains could be applied at scale.
Read: Bring Your Own AI — How to Balance Risks and Innovation
USAA’s lessons in deploying generative AI internally
At financial services firm USAA, executives have identified multiple internal use cases for pairing employees with AI tools to improve customer service and increase efficiency. (Customer-facing tools aren’t a near-term priority for the company.)
MIT Initiative on the Digital Economy fellow Thomas H. Davenport and writer Randy Bean examine the company’s efforts in a new article. One example is a “copilot” to help member service representatives find information, answer questions, and summarize interactions with customers across various channels. Another is an AI-powered code development system that works alongside human programmers to assist with documentation, testing, and other tasks.
To ease employees’ concerns that AI — or new workers with AI skills — will replace them, USAA executives have committed to supporting their current workforce. This involves expanding training options, improving productivity for current workers, and emphasizing the long-term value of problem-solving skills that employees have built through years of experience.
Read: How Gen AI Helps USAA Innovate
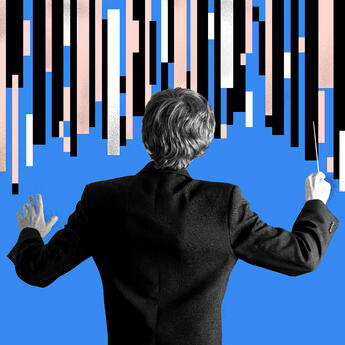
Leading the AI-Driven Organization
In person at MIT Sloan
Register Now
How to ensure that LLMs work in the enterprise
A large language model is a generative AI system trained on a large amount of text data to craft contextually appropriate answers to inputs. MIT Sloan professor of the practice writes that LLMs are powerful but prone to error. As a result, gaining value from LLMs in an enterprise setting requires that those models be optimized to ensure that their output as accurate as possible.
The first step is to adapt the LLM to a specific task. Simple instructions, or prompts, may work if the task is one that a layperson could accomplish. Retrieval-augmented generation can add updated information (including proprietary data) to a prompt to elicit a more accurate response. Instruction fine-tuning goes further and brings domain-specific examples into the LLM itself; this can be helpful for legal or health care models, but it requires some heavy lifting.
Next, organizations need to determine when it makes sense to apply LLMs to a task. This is a matter of breaking a business process into discrete tasks and comparing the cost of using an LLM to accomplish a task to the cost of “business as usual.” Critically, this calculation must consider the potential cost of errors in the output, whether it’s legal liability, reputational risk, or brand damage.
Finally, organizations should be ready to pilot LLM use cases and evaluate their effectiveness. This can be difficult to automate, as text responses need to be checked for reasoning, tone, and relevance — factors absent from AI models trained to output sets of numbers.
Read: A Practical Guide to Gaining Value from LLMs
6 steps for forging collaborations built on AI
As firms continue to explore AI use cases, it’s worth looking beyond their four walls. Davenport, of the MIT Initiative on the Digital Economy, explores how AI changes partnerships as organizations come together to share data, conduct research, or achieve innovation at a greater scale.
AI typically supports collaboration in four ways: Partners can integrate data ecosystems, add new AI capabilities to existing AI platforms, enhance products and services, and advocate for the responsible development of AI.
Davenport writes that successful partnerships should aim to follow a six-step blueprint:
- Decide what AI will transform; emphasize transformation rather than incremental change.
- Convene a diverse group of collaborators that can accomplish more working together than they could on their own.
- Develop AI-powered services that bring valuable insights to all partners.
- Consider the potential to address broader societal and environmental issues in addition to economic growth.
- Prioritize transparency and robust data protection to maintain trust as partners share sensitive information.
- Embrace flexibility; as technology evolves, so, too, must any collaboration with AI at its core.
Read: How AI Changes Partner Collaboration